Artificial intelligence in psychiatric diagnosis: challenges and opportunities in the era of machine learning
DOI:
https://doi.org/10.25118/2763-9037.2024.v14.1318Keywords:
artificial intelligence, psychiatric diagnosis, machine learning, mental health technology, personalized psychiatryAbstract
The integration of artificial intelligence (AI) into psychiatric diagnosis heralds a new era in mental health care, offering unprecedented opportunities to enhance diagnostic accuracy, personalize treatment, and streamline clinical workflows. A systematic approach was utilized, adhering to the PRISMA (Preferred Reporting Items for Systematic Reviews and Meta-Analyses) guidelines. This literature review explores the current state of AI in psychiatric diagnosis, highlighting key technologies such as machine learning, natural language processing, and deep learning. We discuss the application of these technologies across various psychiatric disorders, including depression, anxiety, and schizophrenia. While AI holds immense promise, significant challenges remain, including issues of data privacy, model bias, and the clinical validation of AI tools. Furthermore, ethical and regulatory considerations must be addressed to ensure responsible implementation. This review also examines the potential future directions of AI in psychiatry, emphasizing the importance of collaboration between AI systems and human clinicians. As the field evolves, AI has the potential to transform psychiatric practice, offering new avenues for early detection, personalized care, and therapeutic monitoring.
Downloads
Metrics
References
Garber M. When PARRY met ELIZA: a ridiculous chatbot conversation From 1972. Washington: The Atlantic; 2014. https://www.theatlantic.com/technology/archive/2014/06/when-parry-met-eliza-a-ridiculous-chatbot-conversation-from-1972/372428/
Lindsay RK, Buchanan BG, Feigenbaum EA, Lederberg J. Applications of artificial intelligence for organic chemistry: the Dendral project. New York: McGraw-Hill; 1980.
Lee EE, Torous J, De Choudhury M, Depp CA, Graham SA, Kim HC, Paulus MP, Krystal JH, Jeste DV. Artificial intelligence for mental health care: clinical applications, barriers, facilitators, and artificial wisdom. Biol Psychiatry Cogn Neurosci Neuroimaging. 2021;6(9):856-64. https://doi.org/10.1016/j.bpsc.2021.02.001 PMID:33571718 - PMCID:PMC8349367 DOI: https://doi.org/10.1016/j.bpsc.2021.02.001
Brunn M, Diefenbacher A, Courtet P, Genieys W. The future is knocking: how artificial intelligence will fundamentally change psychiatry. Acad Psychiatry. 2020;44(4):461-6. https://doi.org/10.1007/s40596-020-01243-8 PMID:32424706 DOI: https://doi.org/10.1007/s40596-020-01243-8
Squires M, Tao X, Elangovan S, Gururajan R, Zhou X, Acharya UR, Li Y. Deep learning and machine learning in psychiatry: a survey of current progress in depression detection, diagnosis and treatment. Brain Inform. 2023;10(1):10. https://doi.org/10.1186/s40708-023-00188-6 PMID:37093301 - PMCID:PMC10123592 DOI: https://doi.org/10.1186/s40708-023-00188-6
AI for Precision Mental Health. Kourtzi Z, Liakata M, organisers; Schönlieb CB, Lyons T, Tino P, researchers. London: The Alan Turing Institute; 2019. https://www.turing.ac.uk/research/research-projects/ai-precision-mental-health
Li M, Jiang Y, Zhang Y, Zhu H. Medical image analysis using deep learning algorithms. Front Public Health. 2023;11:1273253. https://doi.org/10.3389/fpubh.2023.1273253 PMID:38026291 - PMCID:PMC10662291 DOI: https://doi.org/10.3389/fpubh.2023.1273253
Bracher-Smith M, Crawford K, Escott-Price V. Machine learning for genetic prediction of psychiatric disorders: a systematic review. Mol Psychiatry. 2021;26(1):70-9. https://doi.org/10.1038/s41380-020-0825-2 PMID:32591634 - PMCID:PMC7610853 DOI: https://doi.org/10.1038/s41380-020-0825-2
Dwyer DB, Falkai P, Koutsouleris N. Machine learning approaches for clinical psychology and psychiatry. Annu Rev Clin Psychol. 2018;14:91-118. https://doi.org/10.1146/annurev-clinpsy-032816-045037 PMID:29401044 DOI: https://doi.org/10.1146/annurev-clinpsy-032816-045037
Zafar F, Fakhare Alam L, Vivas RR, Wang J, Whei SJ, Mehmood S, Sadeghzadegan A, Lakkimsetti M, Nazir Z. The role of artificial intelligence in identifying depression and anxiety: a comprehensive literature review. Cureus. 2024;16(3):e56472. https://doi.org/10.7759/cureus.56472 DOI: https://doi.org/10.7759/cureus.56472
Shim M, Kim DW, Lee SH, Hwang HJ. Editorial: AI-based computer-aided diagnosis and prognosis for psychiatric disorders. Front Hum Neurosci. 2022;16:901525. https://doi.org/10.3389/fnhum.2022.901525 PMID:35517988 - PMCID:PMC9062226 DOI: https://doi.org/10.3389/fnhum.2022.901525
Ye J, Woods D, Jordan N, Starren J. The role of artificial intelligence for the application of integrating electronic health records and patient-generated data in clinical decision support. AMIA Jt Summits Transl Sci Proc. 2024;2024:459-67. http://www.ncbi.nlm.nih.gov/pmc/articles/pmc11141850/ PMID:38827061 - PMCID:PMC11141850
Perets O, Stagno E, Yehuda EB, McNichol M, Anthony Celi L, Rappoport N, Dorotic M. Inherent bias in electronic health records: a scoping review of sources of bias. medRxiv [Preprint]. 2024:2024.04.09.24305594. https://doi.org/10.1101/2024.04.09.24305594 PMID:38680842 PMCID:PMC11046491 DOI: https://doi.org/10.1101/2024.04.09.24305594
Cresswell K, Rigby M, Magrabi F, Scott P, Brender J, Craven CK, Wong ZS, Kukhareva P, Ammenwerth E, Georgiou A, Medlock S, De Keizer NF, Nykanen P, Prgomet M, Williams R. The need to strengthen the evaluation of the impact of artificial intelligence-based decision support systems on healthcare provision. Health Policy. 2023;136:104889. https://doi.org/10.1016/j.healthpol.2023.104889 PMID:37579545 DOI: https://doi.org/10.1016/j.healthpol.2023.104889
Farah L, Borget I, Martelli N, Vallee A. Suitability of the current health technology assessment of innovative artificial intelligence-based medical devices: scoping literature review. J Med Internet Res. 2024;26:e51514. https://doi.org/10.2196/51514 PMID:38739911 - PMCID:PMC11130781 DOI: https://doi.org/10.2196/51514
Van Laere S, Muylle KM, Cornu P. Clinical decision support and new regulatory frameworks for medical devices: are we ready for it? - A viewpoint paper. Int J Health Policy Manag. 2022;11(12):3159-63. https://doi.org/10.34172/ijhpm.2021.144 PMID:34814678 PMCID:PMC10105190 DOI: https://doi.org/10.34172/ijhpm.2021.144
Karalis VD. The integration of artificial intelligence into clinical practice. Appl Biosci. 2024;3(1):14-44. https://doi.org/10.3390/applbiosci3010002 DOI: https://doi.org/10.3390/applbiosci3010002
Rogers WA, Draper H, Carter SM. Evaluation of artificial intelligence clinical applications: detailed case analyses show value of healthcare ethics approach in identifying patient care issues. Bioethics. 2021;35(7):623-33. https://doi.org/10.1111/bioe.12885 PMID:34046918 DOI: https://doi.org/10.1111/bioe.12885
Pashkov VM, Harkusha AO, Harkusha YO. Artificial intelligence in medical practice: regulative issues and perspectives. Wiad Lek. 2020;73(12 cz 2):2722-7. PMID:33611272 DOI: https://doi.org/10.36740/WLek202012204
Hassan S. Advancing precision psychiatry: leveraging AI and machine learning for personalized diagnosis, treatment, and prognosis. Tustin: MarkTechPost; 2024. https://www.marktechpost.com/2024/07/29/advancing-precision-psychiatry-leveraging-ai-and-machine-learning-for-personalized-diagnosis-treatment-and-prognosis/
IEEE Pulse. Artificial intelligence and the future of psychiatry. IEEE Pulse; 2020. https://www.embs.org/pulse/articles/artificial-intelligence-and-the-future-of-psychiatry/
Lhotská L. Artificial intelligence in medicine and healthcare: opportunity and/or threat. Cas Lek Cesk. 2024;162(7-8):275-8. PMID:38981711
Sanches M. On the unique utility of digital technology for bipolar disorder: is there still room for phenomenology? Bipolar Disord. 2020;22(5):530-1. https://doi.org/10.1111/bdi.12908 PMID:32276283 DOI: https://doi.org/10.1111/bdi.12908
Bajwa J, Munir U, Nori A, Williams B. Artificial intelligence in healthcare: transforming the practice of medicine. Future Healthc J. 2021;8(2):e188-94. https://doi.org/10.7861/fhj.2021-0095 PMID:34286183 - PMCID:PMC8285156 DOI: https://doi.org/10.7861/fhj.2021-0095
DigitalDefynd. 40 detailed artificial intelligence case studies. [place unknown]: DigitalDefynd; c2024. https://digitaldefynd.com/IQ/artificial-intelligence-case-studies/
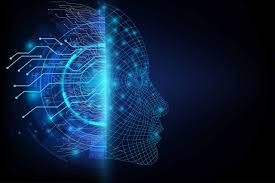
Published
How to Cite
Conference Proceedings Volume
Section
License
Copyright (c) 2024 Kirolos Eskandar

This work is licensed under a Creative Commons Attribution-NonCommercial 4.0 International License.
Debates em Psiquiatria allows the author (s) to keep their copyrights unrestricted. Allows the author (s) to retain their publication rights without restriction. Authors should ensure that the article is an original work without fabrication, fraud or plagiarism; does not infringe any copyright or right of ownership of any third party. Authors should also ensure that each one complies with the authorship requirements as recommended by the ICMJE and understand that if the article or part of it is flawed or fraudulent, each author shares responsibility.
Attribution-NonCommercial 4.0 International (CC BY-NC 4.0) - Debates em Psiquiatria is governed by the licencse CC-By-NC
You are free to:
- Share — copy and redistribute the material in any medium or format
- Adapt — remix, transform, and build upon the material
The licensor cannot revoke these freedoms as long as you follow the license terms. Under the following terms:
- Attribution — You must give appropriate credit, provide a link to the license, and indicate if changes were made. You may do so in any reasonable manner, but not in any way that suggests the licensor endorses you or your use.
- NonCommercial — You may not use the material for commercial purposes.
No additional restrictions — You may not apply legal terms or technological measures that legally restrict others from doing anything the license permits.